Jingming Wang
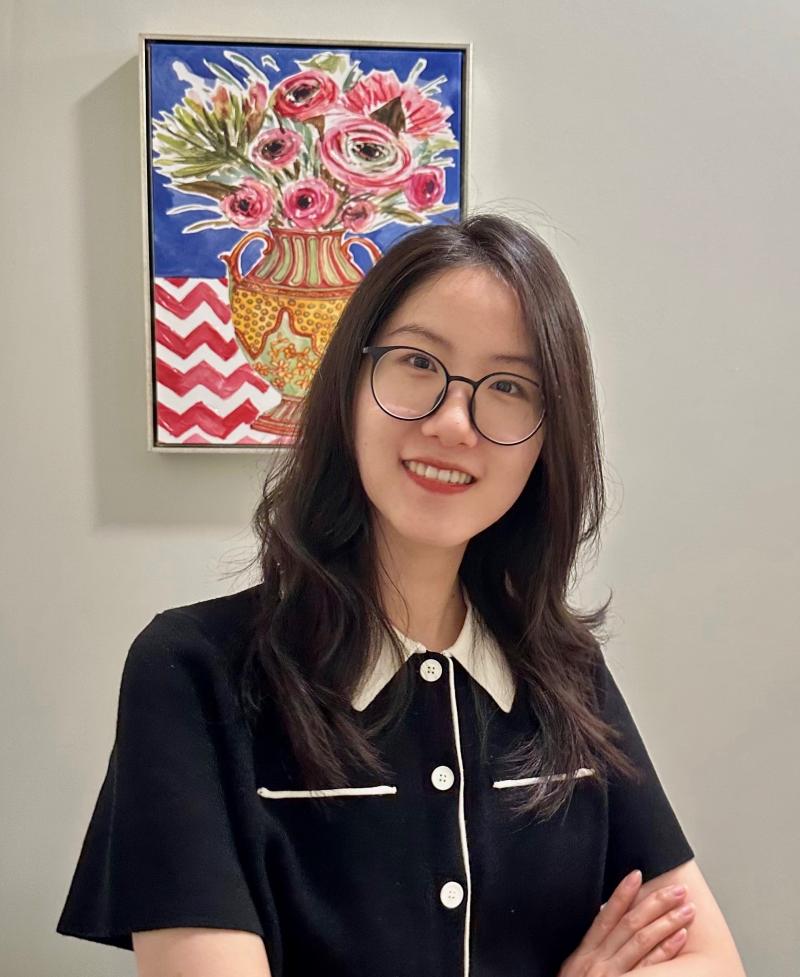
As a statistician with interests in theoretical analysis and motivation from practical applications, Jingming Wang focuses primarily on addressing high-dimensional problems in complex data using random matrix theory and spectral methods. Her recent research topics include network analysis, topic modeling and high-dimensional clustering.
Wang has published her work in leading journals like the Annals of Statistics and at meetings such as the International Conference on Learning Representations. During her Ph.D. studies, she was awarded the Hong Kong Fellowship Scheme by the Hong Kong Research Grants Council. She also won the HKSS-John Aitchison Prize in Statistics — awarded by the Hong Kong Statistical Society — for her excellent Ph.D. research in statistics.
Wang received her Ph.D. in mathematics from the Hong Kong University of Science and Technology in 2021 where she focused on probability and statistics, and prior to joining the faculty at UVA, she was a postdoctoral fellow in the Department of Statistics at Harvard University.
In her first year at UVA, Wang will continue her research in network analysis from both theoretical and practical perspectives. Additionally, she will explore the applications of random matrix theory in machine learning problems, with a particular emphasis on variations of sample covariance matrix models. This fall, she will be teaching introductory courses in mathematical statistics.